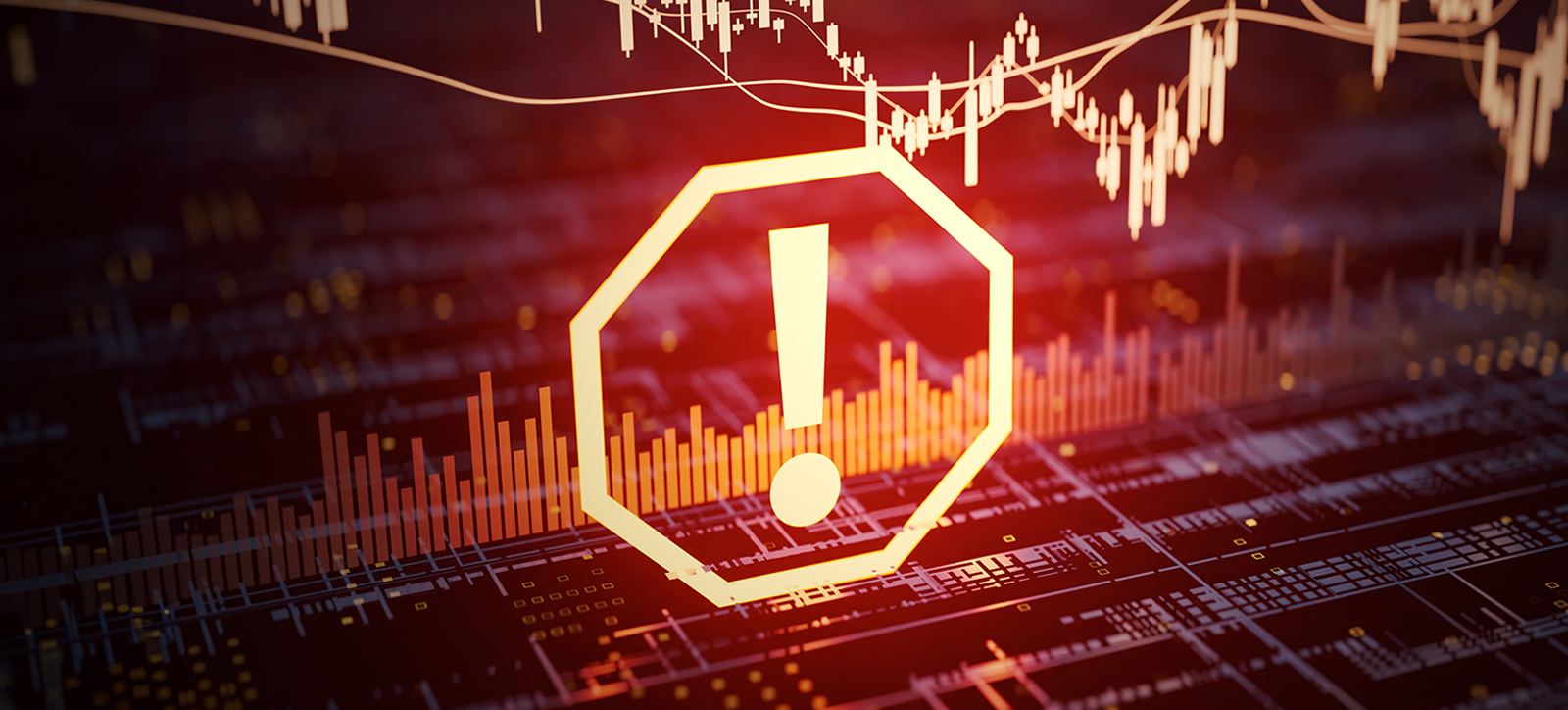
Fatal flaw: The failure of a widely used investment model underlines the challenges in predicting corporate bond returns
Two years ago, I was approached by one of our then-PhD students, Alexander Dickerson. He had been trying to replicate the findings of an established paper by three Georgetown University professors on priced risk in corporate bonds.
It is a common practice among students to inform their own research and lead to ideas for future papers. However, Dickerson couldn’t get his findings to match those outlined in the paper.
He assumed he was doing something wrong, but we encouraged him to look at other bond databases beyond the one used in the original research. With this information, he was able to replicate his own findings, but again he was unable to reproduce those from the Georgetown paper.
It was the start of a project that led to the publication of a paper by Dickerson, my WBS colleague Philippe Mueller and myself, which has significant ramifications for corporate bond pricing, the asset management industry, and the research sector itself.
It has also won the prestigious FAMA-DFA Prize for the best 2023 paper on capital markets and asset pricing in the Journal of Financial Economics.
Priced risk in corporate bonds
The Georgetown paper by Bai et al in 2019 proposed a four-factor model for predicting returns in the corporate bond market.
Their model added three factors – downside, credit, and liquidity risks – to the bond Capital Asset Pricing Model (CAPM), where the corporate bond market factor is the only source of systematic risk in the bond market. The authors claimed this new four-factor model performed better than the longer-established CAPM, which has been widely criticised in recent years.
However, our research showed that these findings were based on data that contained fundamental errors. There were mistakes around the timelines of the data used. We found that two of the remaining three factors had a ‘lead error’, while the third factor had a ‘lag error’.
In addition, the Bai et al. (2023) proposed factors were heavily and asymmetrically winsorized to limit the value of outlying values in the left tail of the return distribution.
This was done ex-post – a Latin phrase meaning after the fact. In other words, the researchers looked at financial results after they had occurred and used them to predict the likelihood of future returns. This is difficult to justify when it comes to evaluating out-of-sample trading strategies because investors do not have access to future information at the time of portfolio formation.
The ex-post filtering of the left tail leaves the upside potential of the various strategies unchanged while artificially capping their losses over a given holding period. The documented gains from investing in these factors are therefore spurious to some extent.
Once we corrected these errors, we found the heavily-cited multi-factor model provided no incremental pricing ability over the CAPM. Essentially, pricing corporate bonds remains an extremely difficult task because these assets seem to exhibit very little cross-sectional predictability.
How the asset management industry responded to data flaws
The research by Bai et al has now been retracted by the Journal of Financial Economics. This is the first time this has happened. But it has been cited more than 400 times in other papers according to Google Scholar, raising concerns over the validity of those pieces, too.
Factors based on a bond’s downside risk, illiquidity risk, and credit risk had also become part of the investing industry’s practices. As a result, our findings had big implications for quantitative investors and the advice that asset management firms provided to clients.
In general, we had a largely positive reaction from the asset management industry, and many firms thanked us. Others contacted us to point out that investing in corporate bonds remains an attractive and valid concept.
But it’s a disruptive situation for the industry, because the main finding – that it is difficult to outperform the CAPM in corporate bond pricing– makes it harder for asset managers to justify the high fees clients pay them.
Academia quickly realised there was an issue, and was keen to listen to us. Since our paper was published, a new wave of papers has contributed to the corporate bond literature as people seek to create new models and come up with new bond factors/anomalies.
Collectively, it is important to make our research replicable and restore confidence in academic work. On the bright side, more researchers are now working on corporate bond issues.
Do investment strategies work in the corporate bond market?
Recently I have worked with Dickerson – now at UNSW Business School in Sydney – and Yoshio Nozawa from the University of Toronto to produce a novel framework to compute transaction costs of trading strategies using infrequently traded assets.
The main finding here is that, after accounting for transaction costs in the proper way, investment strategies tend not to work any longer.
We demonstrate that if you account for the fact that trading corporate bonds is not always feasible or involves long delays, then the profitability of investing in corporate bonds decreases drastically. This contradicts recent papers in top journals. We take a sample of US active bond mutual funds, and show that just two of the 500 considered funds actually beat the market.
I have also been working with Alex Dickerson and Giulio Rossetti from Warwick Business School to highlight other common pitfalls in the evaluation of corporate bond strategies.
This new project looks at what can go wrong when not properly adjusting for market microstructure noise in transaction-based corporate bond data and when asymmetrically and ex-post winsorizing/trimming the return distribution. We provide a new protocol that is able to handle data uncertainty for any bond signal and database.
Alongside the implications for corporate bonds, our experience has wider implications for academic research.
There is always a pressure for positive findings in academia in order to get papers published. In finance and economics, most papers are empirical, that is, based on data.
It is of the utmost importance to look at data in an unbiased way, without torturing them to say something that may simply not be there. In brief, there is much to be learned also from negative findings.
Further reading:
The iron law in the trillion-dollar FX swaps market has broken. What now?
Did Trump's tweets as President move the currency markets?
How stablecoins will impact foreign exchange markets
Priced risk in corporate bonds
Cesare Robotti is Professor of Finance at Warwick Business School. He teaches Financial Management on the Executive MBA (London) and Quantitative Methods for Finance on the MSc Finance.
Learn more about strategic finance on the on the four-day Executive Education course Strategic Choices at WBS London at The Shard.
For more articles on Finance and Markets sign up to Core Insights here.