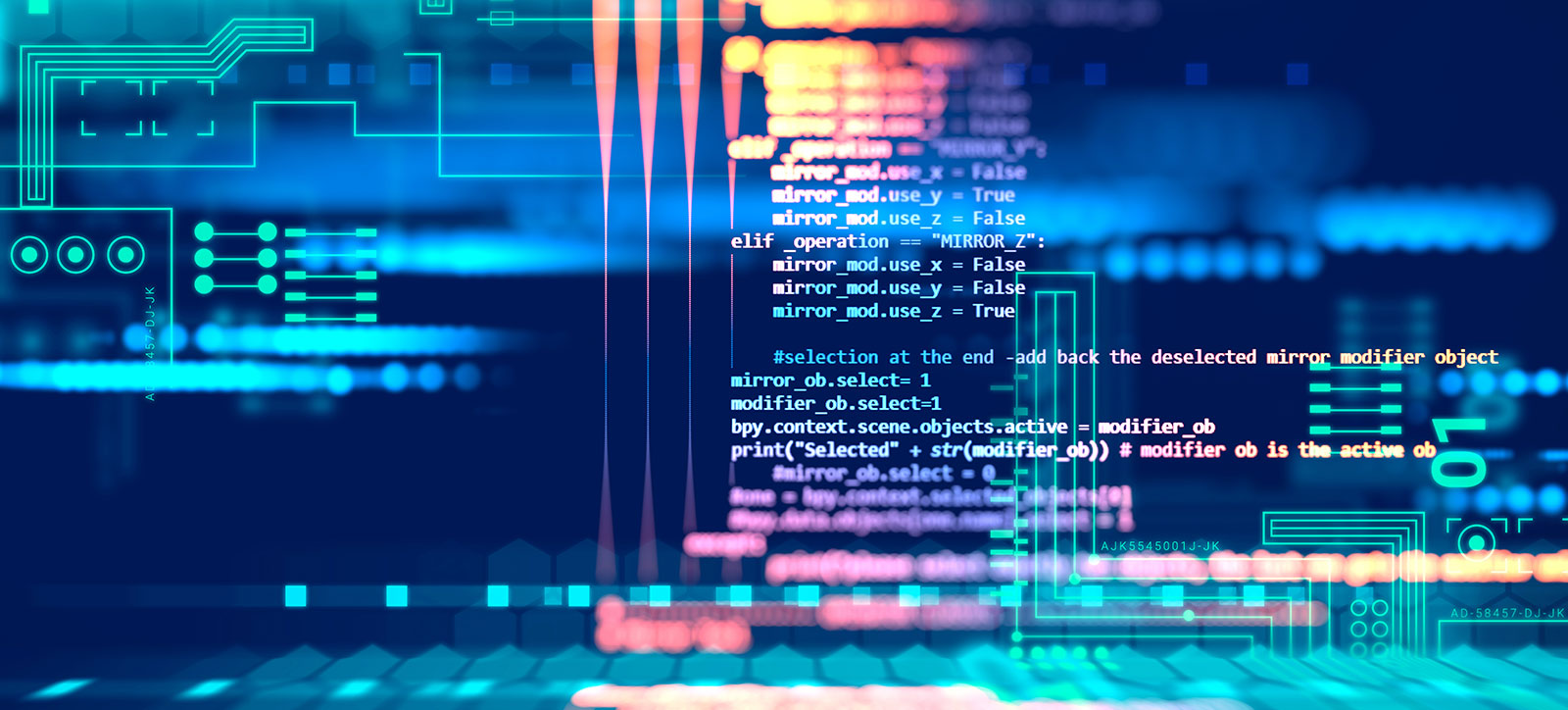
The AIs have it: Those supervising AI need to be trained and incentivised to mitigate AI biases
When you are one of the biggest tech firms in the world, dealing with the tsunami of CVs that every job advert attracts is a huge time-consuming problem.
So, when Amazon developed an artificial intelligence (AI) tool that scoured the thousands of resumes and picked out the top talent for managers to interview, HR bosses thought they were on to an automation winner.
But what they didn’t realise is that after training it on a data set covering 10 years of hiring, the AI model had in effect learned that it was mainly men that were given jobs at Amazon, and so it penalised CVs that included the word 'women's,' such as 'women's chess club captain'.
Reuters revealed it also downgraded graduates of two all-women's colleges. In the end Amazon had to scrap the AI in 2018 and learned a valuable lesson in algorithmic bias.
Automated decisions that discriminate based on race, gender, or age produce sub-optimal outcomes for both the company using the AI – as profitable opportunities can be missed – and for the individuals who are discriminated against. If AI is to be used more widely and effectively, such teething problems need to be ironed out.
Efforts to reduce AI bias in the AI community focus on creating fairer algorithms and higher quality data collection.
But, along with Nanda Kumar and Karl Lang, of Baruch College, our research shows that, in practice, it is not just the performance of the algorithm that is important, but the combined process and outcome of both the AI and the person supervising it.
This both better reflects reality – as most AI is currently supervised by people in some way – and provides a means of mitigating bias in AI.
Human decision-makers tend to have the final say, working alongside the algorithms. But, of course humans suffer from conscious and unconscious biases, which could reinforce rather than adjust the bias in AI systems.
This is why we believe that only when people are channelled by a structured framework focused that is on maximising profit are they actually an effective means of reducing bias in AI and machine learning.
Our research has found that with a framework in place, over time, human operators working with AI learn to adapt to its biased results by identifying the bias and adjusting their behaviour in response.
This can significantly improve performance, and most importantly, outperform the biased AI working alone in terms of reducing decision bias and increasing organisational profit.
Framework to reduce biases in artificial intelligence
It appears that two brains really are better than one, with human operators able to compensate for the biased algorithm, which also tends to make using AI algorithms more profitable. The effect is not immediate but achieved through repeated review and adjustment.
Staff are provided with feedback on how they and the AI are performing, which enables the identification of biases, so adjustments are made through the human decision-makers’ repeated interactions with the AI or machine learning model.
With repeated exposure, people can learn over time how to overcome any biases in AI or in the data it is using.
All the while, the human operators are working within a corporate framework that aims to minimise bias in final decisions in order to maximise profit.
The framework must include an incentive mechanism, so decision-makers are penalised for approving bad decisions by the AI and gain a bonus for making good ones.
Any bias in this process would lower profits for the company and see the person penalised financially as well, so their behaviour seeks to minimise any bias from the AI irrespective of their personal views.
It could be argued that in some situations bias decisions might be more profitable, which could complicate any corporate decision-making framework, but we believe these are rare.
On the whole corporate goals are centred on ethics or on profitability, and profitability tends to be inversely related to bias, because bias decisions miss profitable opportunities and often fail to maximise market potential.
A real-world example of AI learning bias
The specific example that we looked at in our research was assessing eligibility for bank loans.
If a sizeable number of good loan applicants from under-represented ethnic minorities are rejected, it makes the operation less profitable.
We looked at human decision-makers alone and then AI plus humans, with 50 loan applications in each group.
As they went through the loan applications the human decision-makers were able to learn and overcome the AI bias.
It was the humans that improved, rather than the AI itself, through this process, showing that in many ways people can be more adaptive than AI, at this stage at least.
Of course, we must also reduce the bias in the AI itself and in the data that it is based on, but this is being looked at elsewhere, and there may always be some bias baked in – so teaching humans to correct for this is important.
While the study focused on bank loans, we believe this is transferable to many similar situations involving complex decisions that have a binary good or bad outcome – such as hiring, promoting, or access to medical procedures, as well as access to credit.
All these processes have similar complex structures and compositions, and all result in a binary outcome.
Clearly, our research shows training human operators is important if businesses are to make the most of AI, which means companies need to take a long-term view when integrating AI into their decision-making processes – they need to give employees time to learn and adapt to a new way of working alongside AI.
The approach also needs to be organisation-wide, with careful testing and enough time for training, feedback, and practise in order to get it right.
If organisations put these measures in place they can avoid making costly mistakes like Amazon, something not all companies can easily absorb.
Further reading:
What is responsible artificial intelligence and why do we need it?
Five reads you need to make AI ethical and trustworthy
Anh Luong is Assistant Professor of Business Analytics and teaches Business Analytics on the Undergraduate programme. She also lectures on Advanced Data Analytics on the MSc Business Analytics.
Learn more on how to use data on the four-day Executive Education course Business Analytics for Executives at WBS London at The Shard.
For more articles on Decision Making & Analytics sign up to Core Insights here.